AI Could Help Radiologists Improve Osteoarthritis X-ray Diagnosis
By MedImaging International staff writers
Posted on 25 Oct 2018
Researchers from the Center for Digital Health Innovation at the University of California (San Francisco, CA, USA) have developed a fully automated algorithm for the detection of osteoarthritis with radiographs using the 0–4 Kellgren Lawrence (KL) grading system with a state-of-the-art neural network.Posted on 25 Oct 2018
Osteoarthritis classification in the knee is most commonly done with radiographs using the 0–4 KL grading system where 0 is normal, 1 shows doubtful signs of osteoarthritis, 2 is mild osteoarthritis, 3 is moderate osteoarthritis, and 4 is severe osteoarthritis. KL grading is widely used for clinical assessment and diagnosis of osteoarthritis, usually on a high volume of radiographs, making its automation highly relevant.
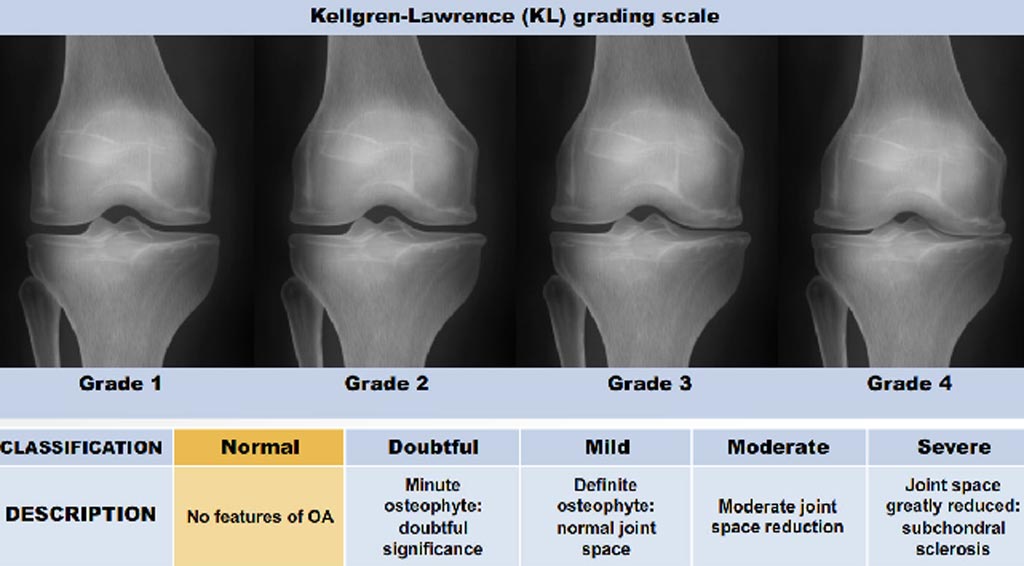
Image: The KL grading system to assess the severity of knee OA. A new UCSF algorithm will help detect OA using this system (Photo courtesy of the University of California, San Francisco).
In order to develop a fully automated algorithm for the detection of osteoarthritis using KL gradings with a state-of-the-art neural network, the researchers collected 4,490 bilateral PA fixed-flexion knee radiographs from the Osteoarthritis Initiative dataset (age = 61.2 ± 9.2 years, BMI = 32.8 ± 15.9 kg/m2, 42/58 male/female split) for six different time points. The left and right knee joints were localized using a U-net model. These localized images were used to train an ensemble of DenseNet neural network architectures for the prediction of osteoarthritis severity.
This ensemble of DenseNets’ testing sensitivity rates of no osteoarthritis, mild, moderate, and severe osteoarthritis were 83.7, 70.2, 68.9, and 86.0%, respectively while the corresponding specificity rates were 86.1, 83.8, 97.1, and 99.1%. Using saliency maps, the researchers confirmed that the neural networks producing these results were in fact selecting the correct osteoarthritic features used in detection. The results suggest that the use of the automatic classifier could assist radiologists in making more accurate and precise diagnosis, given the increasing volume of radiographic image being taken in clinics.
Related Links:
University of California