Google Tests AI Algorithm to Help Detect Metastatic Breast Cancers
By MedImaging International staff writers
Posted on 25 Oct 2018
Scientists at Google AI (Mountain View, CA, USA) who have been developing an algorithm to detect the spread of breast cancer have published new research showing its promise as an assistive tool for pathologists.Posted on 25 Oct 2018
Google AI had described its deep learning–based approach to improve diagnostic accuracy (LYmph Node Assistant, or LYNA) to the 2016 ISBI Camelyon Challenge, which provides gigapixel-sized pathology slides of lymph nodes from breast cancer patients for researchers to develop computer algorithms to detect metastatic cancer. LYNA has achieved significantly higher cancer detection rates than had been previously reported. In their latest published studies, the scientists presented a proof-of-concept pathologist assistance tool based on LYNA and their investigation of these factors.
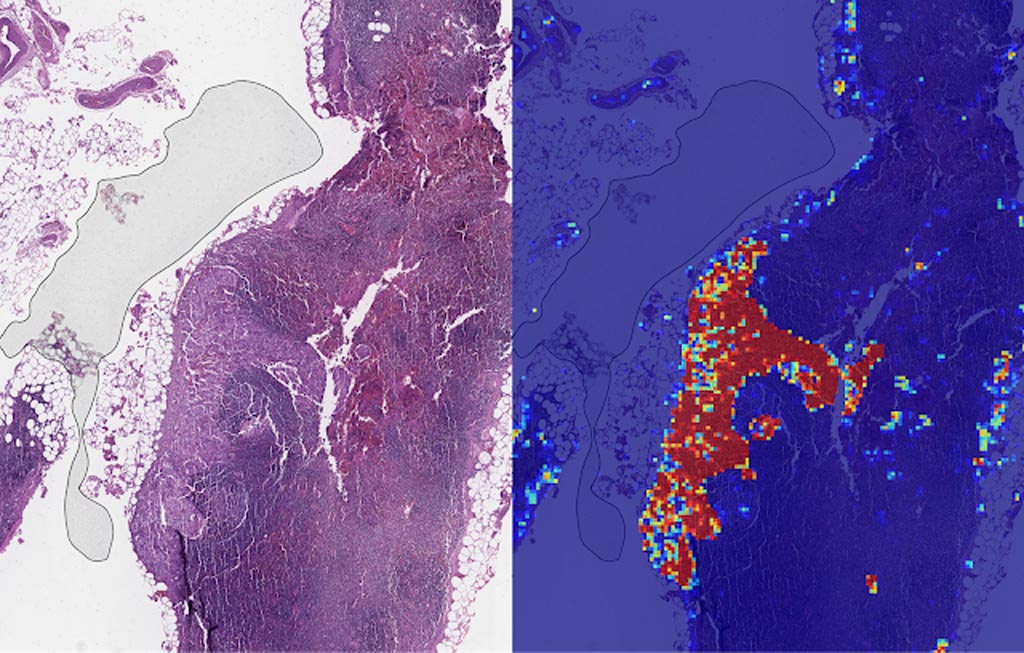
Image: Left: sample view of a slide containing lymph nodes, with multiple artifacts: the dark zone on the left is an air bubble, the white streaks are cutting artifacts, the red hue across some regions are hemorrhagic (containing blood), the tissue is necrotic (decaying), and the processing quality was poor. Right: LYNA identifies the tumor region in the center (red), and correctly classifies the surrounding artifact-laden regions as non-tumor (blue) (Photo courtesy of Google AI).
In the first paper, the scientists applied their algorithm to de-identified pathology slides from both the Camelyon Challenge and an independent dataset provided by our co-authors at the Naval Medical Center San Diego. This additional dataset consisted of pathology samples from a different lab using different processes, thereby improving the representation of the diversity of slides and artifacts seen in routine clinical practice. LYNA proved robust to image variability and numerous histological artifacts, and achieved similar performance on both datasets without additional development.
In both the datasets, LYNA was able to correctly distinguish a slide with metastatic cancer from a slide without cancer 99% of the time. Additionally, LYNA could accurately pinpoint the location of both cancers and other suspicious regions within each slide, some of which were too small to be consistently detected by pathologists. Based on this, the researchers believe that one potential benefit of LYNA could be to highlight these areas of concern for pathologists to review and determine the final diagnosis.
In their second paper, six board-certified pathologists completed a simulated diagnostic task in which they reviewed lymph nodes for metastatic breast cancer both with and without the assistance of LYNA. For the often-laborious task of detecting small metastases (termed micrometastases), the use of LYNA made the task subjectively “easier” (according to pathologists’ self-reported diagnostic difficulty) and halved average slide review time, requiring about one minute instead of two minutes per slide.
This indicates the intriguing potential for assistive technologies such as LYNA to reduce the burden of repetitive identification tasks and to allow more time and energy for pathologists to focus on other, more challenging clinical and diagnostic tasks. In terms of diagnostic accuracy, pathologists in this study were able to more reliably detect micrometastases with LYNA, reducing the rate of missed micrometastases by a factor of two. Encouragingly, pathologists with LYNA assistance were more accurate than either unassisted pathologists or the LYNA algorithm itself, indicating that people and algorithms can work together effectively to perform better than working independently.
Related Links:
Google AI