ML Model Combines Imaging, Clinical, and DNA Methylation Biomarkers for Early Lung Cancer Detection
Posted on 17 Aug 2023
Lung cancer is responsible for a significant number of cancer-related deaths around the globe. Although various treatments, including chemotherapy, immunotherapy, and surgery, have progressed, the overall outlook for lung cancer patients remains grim. This mainly stems from late diagnosis, often at stages III or IV, when the five-year survival rate falls below 10%. Early detection at stages 0–II could significantly lower mortality, but the lack of sensitive technologies and noticeable symptoms in early stages presents substantial challenges.
Deoxyribonucleic acid (DNA) methylation biomarkers have shown potential for early lung cancer detection, as they indicate events connected to tumor initiation. The use of next-generation sequencing methods to identify methylation patterns in circulating tumor DNA could enable non-invasive lung cancer screening. While low-dose computerized tomography (LDCT) has been effective in early detection among high-risk groups, determining the malignancy risk of pulmonary nodules via LDCT remains a challenge. Now, researchers have developed and validated a combined machine learning model comprising imaging, clinical, and cell-free DNA methylation biomarkers that improves the classification of pulmonary nodules and enables earlier diagnosis of lung cancer.
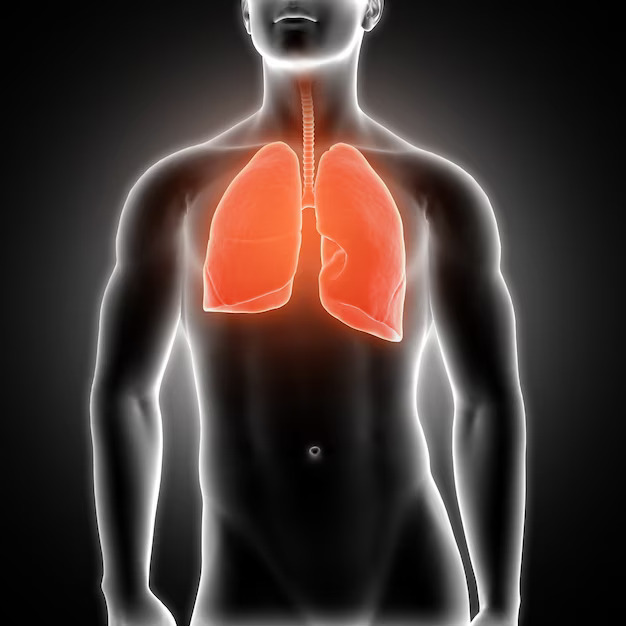
In the new study, researchers at Guangzhou Medical University (Guangzhou, China) developed a combined model of clinical and imaging biomarkers (CIBM) that uses machine learning algorithms to differentiate malignant and benign pulmonary nodules. When integrated with PulmoSeek, a pre-existing cell-free DNA methylation model, the CIBM model can identify small-sized nodules to diagnose lung cancer in its initial stages. For their study, the researchers conducted a study involving participants 18 years and older, with specific types of pulmonary nodules, across 20 Chinese cities. Utilizing over 800 samples, the researchers trained the machine-learning algorithm of the CIBM model to distinguish between benign and malignant tumors. This CIBM model was then integrated with PulmoSeek to create PulmoSeek Plus, a combined diagnostic model. Using decision curve analysis, the team evaluated its clinical application, classifying nodules into risk groups. The aim was to evaluate the performance and diagnostic ability of three models: PulmoSeek, CIBM, and PulmoSeek Plus.
The results showed that PulmoSeek Plus holds the potential for successful early-stage diagnosis of benign or malignant pulmonary nodules. Used in conjunction with LDCT, this model could be a powerful tool in the early clinical evaluation of lung cancer. The combination of CIBM with the PulmoSeek model heightened the sensitivity of nodule classification by 6% and the negative predictive value by 24%. Moreover, the model’s performance remained strong across different types, sizes, and stages of pulmonary nodules, with sensitivities of characterization for early-stage and small nodules at 0.98 and 0.99, respectively. Particularly noteworthy was its 100% characterization sensitivity for sub-solid nodules, which are typically hard to categorize using LDCT alone. The creation of the PulmoSeek Plus model marks a significant advancement in early lung cancer detection. Given its sole requirement of non-invasive blood samples and CT images, the model offers an efficient and promising approach that could fundamentally change how lung cancer is diagnosed and managed.
Related Links:
Guangzhou Medical University