CT AI Algorithm Categorizes Lung Nodule Cancer Risk
By MedImaging International staff writers
Posted on 20 May 2020
A new study indicates that an artificial intelligence (AI) strategy can correctly assess and categorize suspicious indeterminate pulmonary nodules (IPNs). Posted on 20 May 2020
Developed at Vanderbilt University (Nashville, TN, USA), Optellum (Oxford, United Kingdom), and other institutions, the lung cancer prediction convolutional neural network (LCP-CNN) model was first trained using computerized tomography (CT) images of IPNs from the U.S. National Lung Screening Trial (NLST), internally validated, and externally tested on cohorts from two academic institutions. The researchers then compared the LCP-CNN to traditional risk prediction models on a very large dataset of 15,693 nodules.
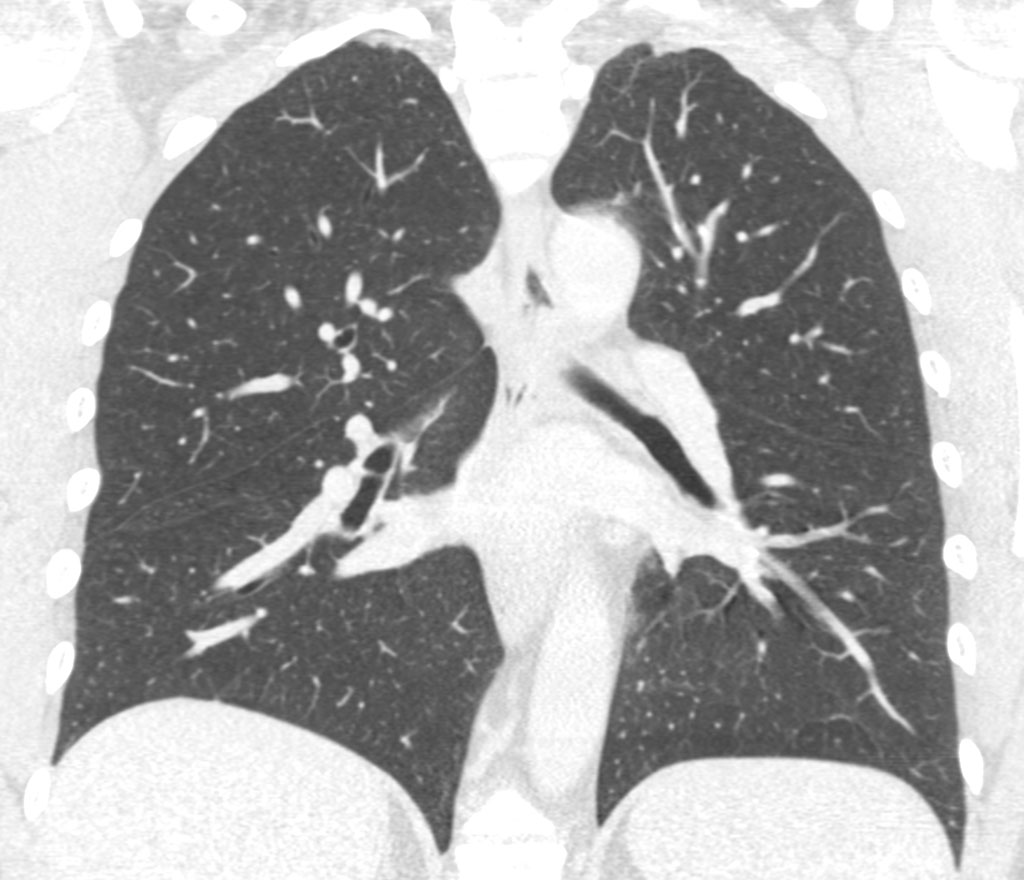
Image: Indeterminate pulmonary nodules on a lung CT (Photo courtesy of Optellum)
The results showed that the AI risk model was associated with an improved accuracy in the predicted disease risk calculation at each threshold of therapy management, as well as in the external validation cohorts. When compared to conventional risk models currently used, the LCP-CNN algorithm reclassified the IPNs into low-risk or high-risk categories in over a third of cancers and benign nodules. The study was published on April 24, 2020, in the American Journal of Respiratory and Critical Care Medicine.
“The management IPNs remains challenging, and strategies to decrease the rate of unnecessary invasive procedures and to optimize surveillance regimens are needed,” concluded lead author Professor Pierre Massion, MD, of Vanderbilt University, and colleagues. “This study demonstrates that this deep learning algorithm can correctly reclassify IPNs into low or high-risk categories, potentially reducing the number of unnecessary invasive procedures and delays in diagnosis.”
Deep learning is part of a broader family of AI machine learning methods that use data representations, as opposed to task specific algorithms. It involves CNN algorithms that execute a cascade of many layers of nonlinear processing units in order to enable feature extraction, conversion, and transformation. Each successive layer uses the output from the previous layer as input to form a hierarchical representation.
Related Links:
Vanderbilt University
Optellum