AI Module Delivers Predictive Image Segmentation and Processing
By MedImaging International staff writers
Posted on 23 Dec 2019
A powerful image analysis and processing module leverages deep learning and artificial intelligence (AI) to accurately extract unbiased data from vast amounts of microscopy datasets.Posted on 23 Dec 2019
The Nikon Instruments (Melville, NY, USA) NIS.ai microscopy image analysis and processing module is a suite of AI-based processing tools that utilizes convolutional neural networks (CNNs) in order to learn how to read images from small training datasets supplied by the user. The training results can then be applied to process and analyze huge volumes of data, allowing researchers to increase throughput and expand their application limits. The NIS.ai includes a suite of applications for predictive imaging, image segmentation, and processing. These include:
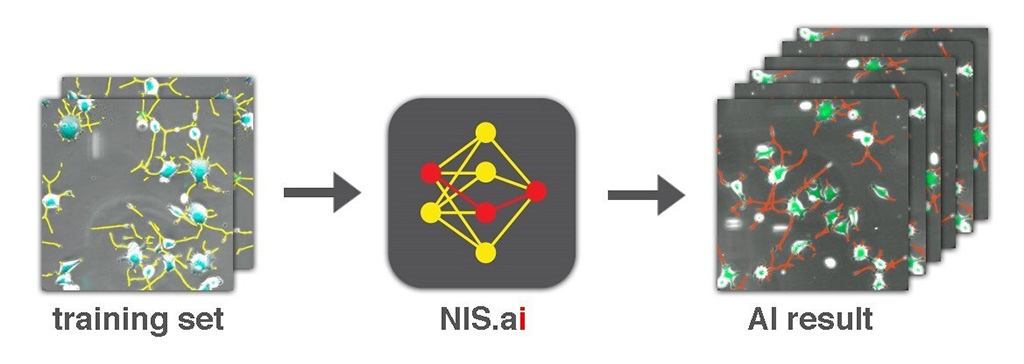
Image: A suite of microscopy applications aid predictive imaging, segmentation, and processing (Photo courtesy of Nikon Instruments)
Convert.ai, which learns related patterns in two different imaging channels. After training, Convert.ai can predict the pattern in the second channel, even when presented with only the first channel. It can also be trained to predict where DAPI-based fluorescent staining of nuclei--a common method for cell segmentation and counting--could be based on unstained differential interference contrast (DIC) or phase-contrast microscopy images. This enables users to perform nuclei-based image analysis without ever having to stain samples with DAPI or acquire a fluorescent channel.
Segment.ai, which enables complex structures to be easily identified and segmented. Neurites in phase-contrast images are traditionally difficult to define by classic thresholding. Segment.ai can be trained on a small subset of hand-traced neurites to automatically detect and segment neurites from thousands of untraced datasets.
Enhance.ai, which allows dim fluorescent samples with poor signal-to-noise ratio (SNR) to be enhanced by learning what a high signal-to-noise image looks like, via a process that compares under-exposed and optimally-exposed images. Enhance.ai can then restore details in under-exposed or dim fluorescent images, enabling researchers to gain more insights from their low-signal imaging applications.
Denoise.ai, which removes shot noise from resonant confocal images and can be performed in real-time. Applying Denoise.ai to resonant confocal imaging enables users to acquire confocal images at ultra-high speed without sacrificing image quality.
“The application of Deep Learning and AI to biomedical imaging is extremely powerful, and opening up unseen possibilities,” said Steve Ross, PhD, director of products and marketing at Nikon Instruments. “With NIS.ai, researchers can easily apply deep learning to extract meaningful, unbiased data from large, complex datasets.”
Related Links:
Nikon Instruments