Artificial Neural Network Improves Prostate Cancer Detection
By MedImaging International staff writers
Posted on 29 Apr 2019
A new artificial intelligence (AI) system identifies and predicts the aggressiveness of prostate cancer (PC) at the same level of accuracy as experienced radiologists.Posted on 29 Apr 2019
Developed at the University of California, Los Angeles (UCLA; USA), FocalNet is a convolutional neural network (CNN) that uses an algorithm with more than a million trainable variables. The CNN was trained using multi-parametric MRI (mp-MRI) scans of 417 men with PC prior to robotic-assisted laparoscopic prostatectomy (RALP). In order to learn how to classify the aggressiveness of the tumor using the Gleason score (GS), the results were compared to the actual pathology specimen. They then compared the AI system's results with readings by UCLA radiologists who had more than 10 years of experience.
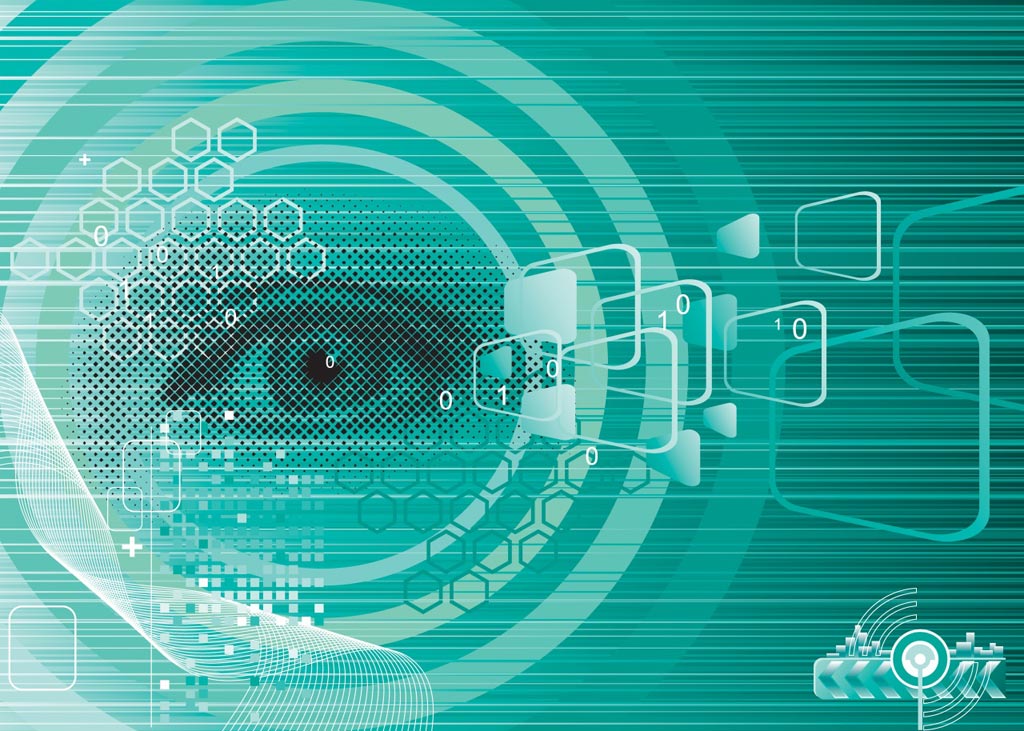
Image: New research hints that AI may soon make radiologists redundant (Photo courtesy of 123rf.com).
The results revealed that in the free-response receiver operating characteristics (FROC) analysis for lesion detection, FocalNet showed 89.7% and 87.9% sensitivity for index lesions and clinically significant lesions, respectively. With the comparison to the prospective performance of radiologists using current diagnostic guidelines, FocalNet demonstrated a detection sensitivity for clinically significant lesions (80.5%) comparable to that of radiologists with at least 10 years of experience (83.9%). The study was presented at the IEEE International Symposium on Biomedical Imaging (ISBI), held during April 2019 in Venice (Italy).
“Multi-parametric MRI is considered the best non-invasive imaging modality for diagnosing prostate cancer. However, mp-MRI for PC diagnosis is currently limited by the qualitative or semi-quantitative interpretation criteria, leading to inter-reader variability and a suboptimal ability to assess lesion aggressiveness,” concluded senior author Kyunghyun Sung, of the UCLA department of radiology, and colleagues. “CNNs are a powerful method to automatically learn the discriminative features for various tasks, including cancer detection.”
CNN’s use a cascade of many layers of nonlinear processing units for feature extraction and conversion, with each successive layer using the output from the previous layer as input to form a hierarchical representation.
Related Links:
University of California, Los Angeles