Radiomics Predicts Patient Benefits from Chemotherapy
By MedImaging International staff writers
Posted on 04 Apr 2019
Data retrieved from computed tomography (CT) images may be able to forecast which lung cancer patients will respond to chemotherapy, claims a new study.Posted on 04 Apr 2019
Researchers at Case Western Reserve University (CWRU; Cleveland, OH, USA), the Cleveland Clinic (CC; OH, USA), and other institutions retrospectively analyzed data from 125 patients with non–small cell lung cancer (NSCLC) who had been treated with pemetrexed-based platinum doublet chemotherapy, in order to identify the role of radiomics texture features--both within and outside the nodule--in predicting time to progression (TTP), overall survival (OS), and response to chemotherapy.
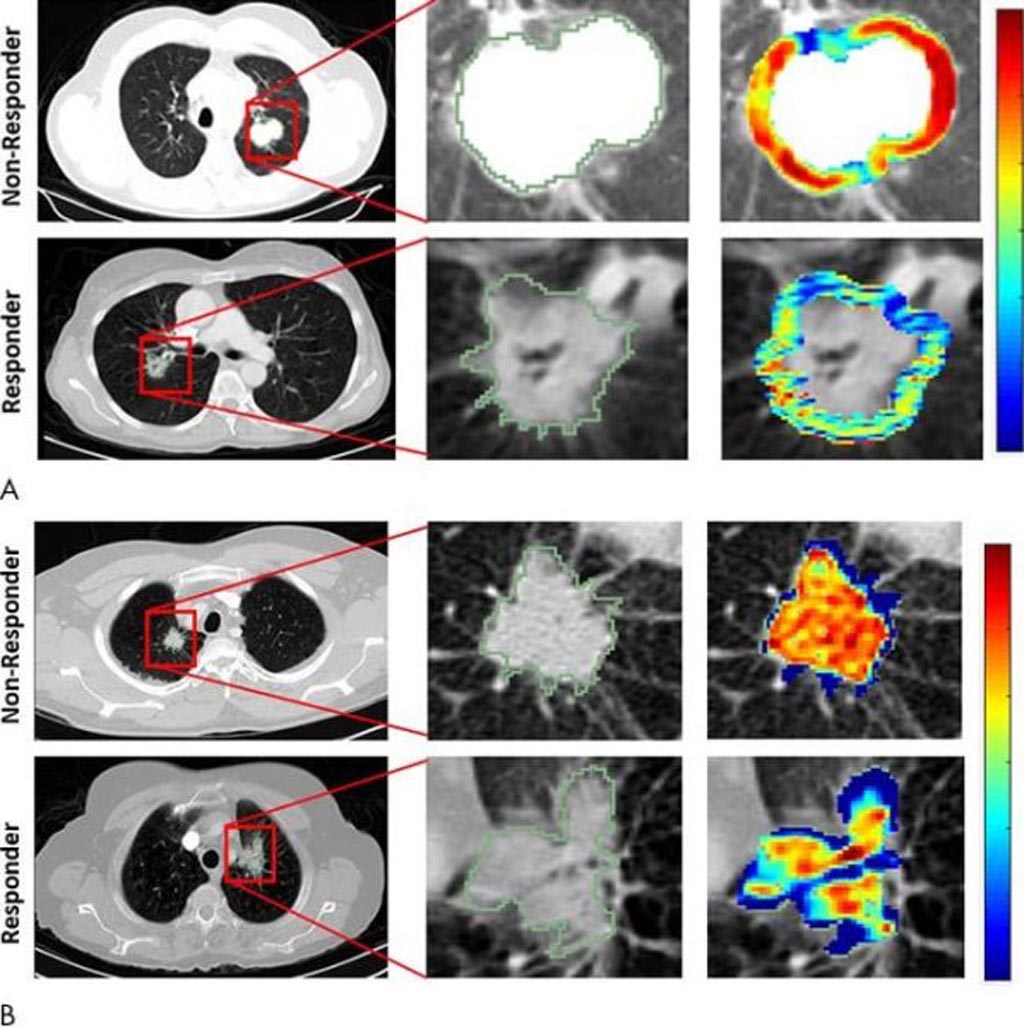
Image: Radiomic feature maps show differences between lesions in responders and nonresponders at pretreatment CT (Photo courtesy of Cleveland Clinic).
The patients were divided randomly into two sets, with the constraint that there were an equal number of responders and nonresponders in the training set. The training set comprised 53 patients with NSCLC, and the validation set comprised 72 patients. A machine learning classifier trained with radiomic texture features extracted from intra- and peritumoral regions of non–contrast-enhanced CT images was used to predict response to chemotherapy. The radiomic risk-score signature was generated by using least absolute shrinkage and selection operator, and association of the radiomic signature with TTP and OS was also evaluated.
The results showed that radiomic features derived from within the tumor and from the area around the tumor were able to distinguish between patients who responded to chemotherapy and those who did not with an accuracy of 0.77. In addition, the radiomic features predicted TTP and OS, and decision curve analysis demonstrated that in terms of clinical usefulness, the radiomics signature had a higher overall net benefit in prediction of high-risk patients to receive treatment than clinical-pathologic measurements. The study was published on March 20, 2019, in Radiology: Artificial Intelligence.
“This is the first study to demonstrate that computer-extracted patterns of heterogeneity, or diversity, from outside the tumor were predictive of response to chemotherapy,” said co-lead author Monica Khunger, MD, of the CC. “This is very critical because it could allow for predicting in advance of therapy which patients with lung cancer are likely to respond or not. This could help identify patients who are likely to not respond to chemotherapy for alternative therapies such as radiation or immunotherapy.”
Radiomics is an extension of computer-aided diagnosis, and refers to the comprehensive quantification of tumor phenotypes by extracting a large number of quantitative image features for data mining and precision medicine. In recent years radiomics has successfully extracted a variety of clinically relevant features, merging them into signatures, in order to estimate the probability of malignancy of identified breast cancer lesions.
Related Links:
Case Western Reserve University
Cleveland Clinic