Imaging Software Analyzes Kidney Stone Composition
By MedImaging International staff writers
Posted on 26 Dec 2017
A streamlined, semi-automated tool analyzes kidney stones instantly using a patient’s computerized tomography (CT) scans.Posted on 26 Dec 2017
The StoneChecker (Radstock, United Kingdom) software, based on the TexRAD (Cambridge, United Kingdom) algorithm, examines the physical attributes of a renal stone on non-contrast enhanced CT scan slices. The patented filtration-histogram CT Texture Analysis (CTTA) algorithm highlights coarse features, using histogram analysis to quantify and assess distribution of grey-levels, coarseness, and regularity within the region of interest. Filters that extract and enhance image features at larger scales reduce noise artifacts, whilst heterogeneity in stone architecture is enhanced.
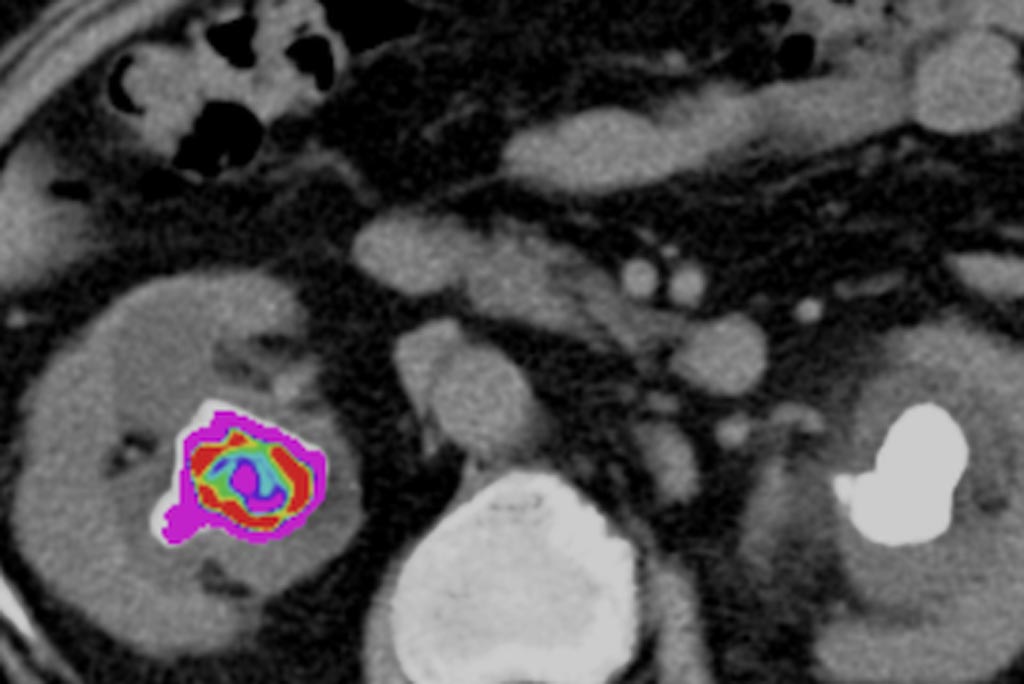
Image: CT texture metrics can identify kidney stone composition (Photo courtesy of StoneChecker).
Stonechecker then prepares a report of a stone’s given characteristics, such as volume, mean Hounsfield unit (HU) density, skin to stone distance, entropy, kurtosis, skew and other metrics. Each of these factors has been shown to be relevant in predicting stone-free rates and the potential outcome of a lithotripsy procedure carried out on the stone. Heterogeneity parameters at different spatial scales enable quantitative assessment of imaging biomarkers within the kidney stones, which can then be used to identify treatment failure on pre-treatment CT.
“CTTA metrics reflect stone characteristics and composition and predict ease of shock wave lithotripsy fragmentation. The strongest correlation with the number of shocks required to fragment a kidney stone is mean HU density and the entropy of the pixel distribution of the stone image,” reports the company. “With the aid of multiple linear regression analysis, the CTTA metrics of entropy and kurtosis can predict 92% of the outcome of number of shocks needed to fragment the stone.”
Kidney stones are often no larger than a grain of rice, yet some can grow to a diameter of several centimeters, causing blockage of the ureters. If it cannot be dissolved chemically, the kidney stone is treated using extracorporeal shock-wave therapy or minimally invasive endoscopic modalities. Many of these patients suffer from disease recurrence and need retreatment, but new stone formation might be reduced by adapting dietary habits or the use of particular medication strategies, as based on stone composition.
Related Links:
StoneChecker
TexRAD