Deep Learning Based Algorithms Improve Tumor Detection in PET/CT Scans
Posted on 03 Jan 2025
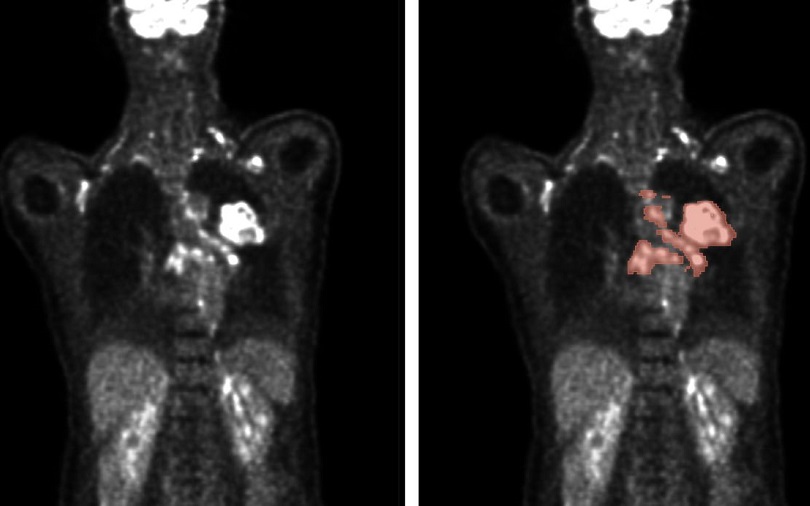
Imaging techniques are essential for cancer diagnosis, as accurately determining the location, size, and type of tumors is critical for selecting the appropriate treatment. The key imaging methods include positron emission tomography (PET) and computed tomography (CT). PET utilizes radionuclides to visualize metabolic activity within the body, with malignant tumors exhibiting significantly higher metabolic rates than benign tissues. For this, fluorine-18-deoxyglucose (FDG), a radioactively labeled glucose, is commonly employed. In CT, the body is scanned layer by layer using an X-ray tube to visualize anatomical structures and pinpoint tumors. Cancer patients often present with numerous lesions—pathological changes resulting from tumor growth—and capturing all lesions for a comprehensive view is necessary. Typically, doctors manually mark tumor lesions on 2D slice images, a process that is very time-consuming. An automated algorithm for evaluation could drastically reduce time and enhance diagnostic accuracy.
In 2022, researchers from the Karlsruhe Institute of Technology (KIT, Karlsruhe, Germany) participated in the international autoPET competition and placed fifth out of 27 teams, comprising 359 participants globally. autoPET combined imaging with machine learning to automate the segmentation of metabolically active tumor lesions visible on whole-body PET/CT scans. Teams had access to a large, annotated PET/CT dataset to train their algorithms. All final submissions relied on deep learning, a type of machine learning using multi-layered artificial neural networks to identify complex patterns and correlations in large datasets. The seven leading teams from the competition recently shared their findings in the journal Nature Machine Intelligence, highlighting the potential of automated analysis in medical imaging.
The researchers found that an ensemble of the best-performing algorithms outperformed individual models. This ensemble approach allowed for more efficient and accurate detection of tumor lesions. While the algorithm's performance is influenced by the quality and quantity of data, the design of the algorithm, particularly decisions made in post-processing the predicted segmentations, also plays a critical role. The researchers noted that further improvements are needed to enhance the algorithms’ resilience to external factors, with the goal of making them suitable for routine clinical use. The ultimate aim is to fully automate the analysis of PET and CT medical image data in the near future.