AI-Powered Chest X-Ray Analysis Shows Promise in Clinical Practice
Posted on 27 Aug 2024
Recent advancements in artificial intelligence (AI) have fueled interest in computer-assisted diagnosis, driven by growing radiology workloads, a global shortage of radiologists, and the potential for burnout. Radiology departments often encounter a high volume of unremarkable chest X-rays, and AI has the potential to enhance efficiency by automating the reporting process. A new study has demonstrated that off-label use of a commercial AI tool is effective in excluding pathology with equal or fewer critical misses compared to radiologists.
Researchers from Herlev Gentofte Hospital (Copenhagen, Denmark) conducted a study to determine how often AI could accurately identify unremarkable chest X-rays without increasing diagnostic errors. This study analyzed radiology reports and data from 1,961 patients (median age, 72 years; 993 females), each with a single chest X-ray, collected from four Danish hospitals. Previous research indicated that AI tools could confidently exclude pathology in chest X-rays and autonomously generate a normal report. Yet, there was no established threshold for when AI tools should be considered reliable.
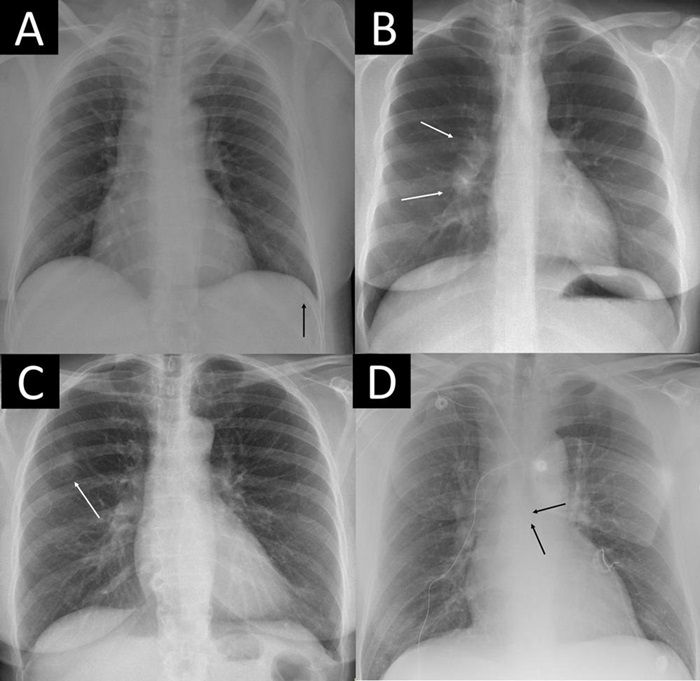
The study aimed to compare the severity of errors made by AI with those made by human radiologists to determine if AI errors were objectively worse. The AI tool calculated a "remarkableness" probability for each X-ray to determine its specificity at various sensitivity levels. Two chest radiologists, blind to AI assessments, categorized the X-rays as "remarkable" or "unremarkable" using established criteria. X-rays with missed findings by either AI or human reports were evaluated by another chest radiologist, who was unaware of who made the error, and classified the misses as critical, clinically significant, or insignificant.
The standard reference found 1,231 of the 1,961 X-rays (62.8%) remarkable and 730 (37.2%) unremarkable. The results, published in the journal Radiology, indicated that the AI tool successfully excluded pathology in 24.5% to 52.7% of unremarkable chest X-rays at sensitivities of 98% or higher, with fewer critical misses than those in the associated radiology reports. However, the mistakes made by AI were generally more severe clinically than those made by radiologists. The study suggested that AI could autonomously report over half of all normal chest X-rays, but emphasized the need for a prospective implementation study of the model at one of the suggested thresholds before recommending widespread use.
Related Links:
Herlev Gentofte Hospital