AI Model Reconstructs Sparse-View 3D CT Scan With Much Lower X-Ray Dose
Posted on 20 Dec 2024
While 3D CT scans provide detailed images of internal structures, the 1,000 to 2,000 X-rays captured from different angles during scanning can increase cancer risk, especially for vulnerable patients. Sparse-view CT scans, which use fewer X-ray projections (as few as 100), significantly reduce radiation exposure but present challenges for accurate image reconstruction. Recently, supervised learning techniques, a form of machine learning that trains algorithms with labeled data, have enhanced the speed and resolution of under-sampled MRI and sparse-view CT image reconstructions. However, labeling large training datasets is both time-consuming and costly. Now, researchers have developed a new framework that works efficiently with 3D images, making the method more applicable to CT and MRI.
This new framework, called DiffusionBlend, was developed by researchers at the University of Michigan Engineering (Ann Arbor, MI, USA). It employs a diffusion model, a self-supervised learning technique that learns a data distribution prior, to enable sparse-view 3D CT reconstruction through posterior sampling. DiffusionBlend learns spatial correlations among nearby 2D image slices, referred to as a 3D-patch diffusion prior, and then blends the scores of these multi-slice patches to create the full 3D CT image volume. When tested on a public dataset of sparse-view 3D CT scans, DiffusionBlend outperformed several baseline methods, including four diffusion approaches at eight, six, and four views, achieving comparable or better computational image quality.
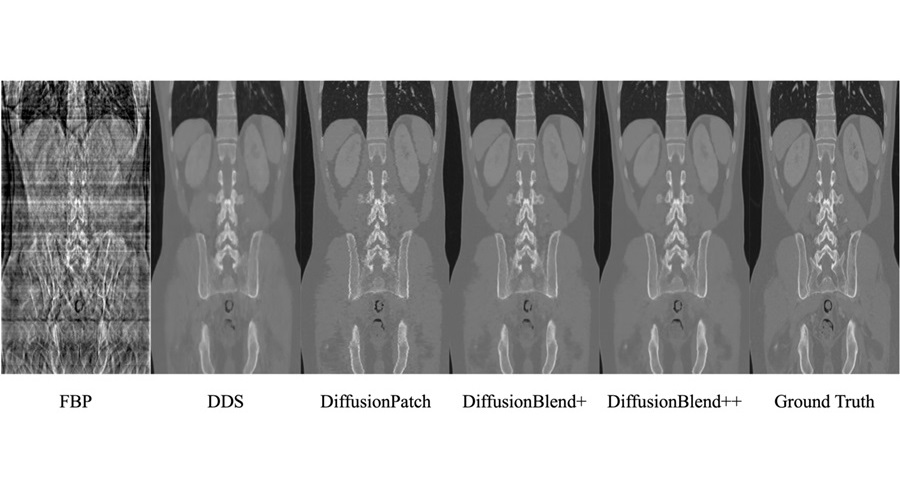
To further enhance its practicality, acceleration methods were applied, reducing DiffusionBlend's CT reconstruction time to one hour, compared to the 24 hours required by previous methods. While deep learning methods can sometimes introduce visual artifacts—AI-generated images of non-existent features—this can be problematic for patient diagnosis. To mitigate this issue, the researchers employed data consistency optimization, specifically using the conjugate gradient method, and evaluated how well the generated images matched the actual measurements using metrics like signal-to-noise ratio.
“We’re still in the early days of this, but there’s a lot of potential here. I think the principles of this method can extend to four dimensions, three spatial dimensions plus time, for applications like imaging the beating heart or stomach contractions,” said Jeff Fessler, the William L. Root Distinguished University Professor of Electrical Engineering and Computer Science at U-M and co-corresponding author of the study.
Related Links:
University of Michigan Engineering